On The Use of Least Expected
Features for 3D Keypoint Detection
by
Isma Hadji
Introduction
Object recognition algorithms rely on the detection of a
subset of important or discriminative visual stimuli (keypoints)
as a first step towards the description of those objects.
Independently of the type of 3D feature used, all 3D detectors
rely on a local criterion for keypoint selection. This criterion
is usually a point-wise saliency measure that is based on
experimentally learnt thresholds. In this research, we question
both the threshold based approach, as well as the local
characteristic of traditional 3D keypoint detection schemes.
First, we abstract the keypoint selection from experimentally
learnt thresholds that depend on low level features for saliency
detection. To this end we propose the Least Expected Feature
criterion (LEFT) for saliency detection. Second we introduce the
concept of finding keypoints considering a global approach, as
opposed to more traditional local neighborhood based approaches.
It turns out that adopting the proposed global LEFT criterion
allows for the selection of very distinctive keypoints across
the entire object, while avoiding sensitive and noisy regions.
Examples of the keypoints detected by the Global LEFT detector
are shown in Figures 1 and 2, and compared to keypoints detected
using the ISS detector. From the figures it is clear that our
criteria only selects outstanding points as opposed to the ISS
detector that selects points along the edges in the pan example,
while it detects keypoints all over the object even in smooth
non-important regions on the dragon example.
For details on the implementation of the proposed LEFT detector,
the experiments and results we refer the reader to our paper in
[1].
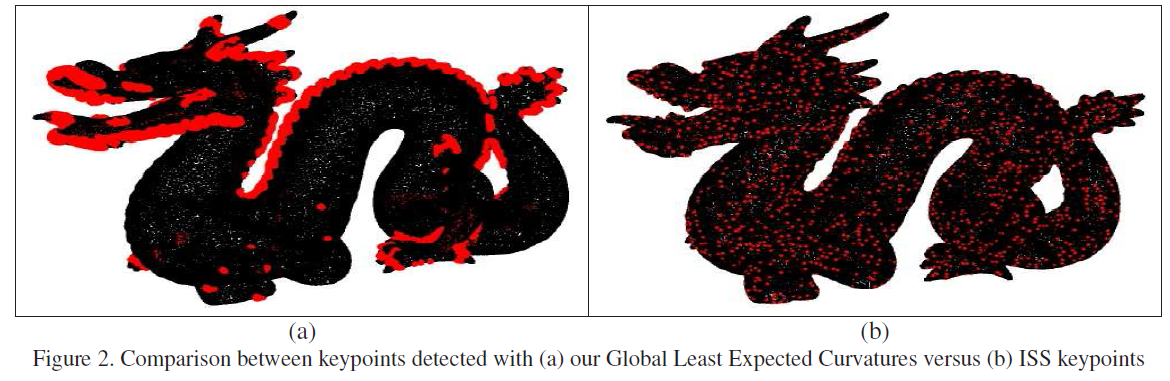
References
-
I. Hadji
and G. N. DeSouza, "On The Use of Least Expected
Features for 3D Keypoint Detection ". (Under review for
WACV 2015)
|